The great philosopher David Hume wrote that “Reason is, and ought only to be, the slave of the passions, and can never pretend to any other office than to serve and obey them” [1]: emotion is the force that propels thought and action.
To understand Hume’s thesis, think about what happens when a kid asks you “why?” over and over again. Why you wake up in the morning, why go to work every day, why you need money, why you want to buy tickets to the Westminster Dog Show—your answer is eventually something about what brings you amusement, joy, or satisfaction, and what leaves you sad, angry, or regretful. And your next answer, if you’re familiar with the state of emotion science, will be that “there’s no scientific consensus about that yet.”
If emotions are so fundamental, why is there so little scientific consensus about them? Emotion scientists can’t seem to agree on answers to some of the most basic questions about emotion. Why do some people find dogs amusing? Why do we seek “amusement” out? Is “amusement” an evolved response, shared by all humans? Is it culture-specific?
In this Spotlight, I will discuss how I have sought out more definite answers to these questions. When I began on this journey around ten years ago, it was as a skeptical data scientist. I quickly saw that scientists with different theories of emotion were interpreting the same data in radically different ways. And the problem wasn’t with the theories, I concluded—it was with the data. The existing data were often too small, too constrained, to provide unequivocal support to any of the complex theories that had become popular in the field.
I began to look for answers using richer, broader, more open-ended approaches, and found that new tools—the internet, large-scale statistics, machine learning—offered new possibilities for understanding human emotion. This marked a turning point on a journey that would take me from improv clubs to museums to record emotional expressions in audiences and ancient sculptures, to the offices of big tech companies, where I advised teams seeking to build technology that accounts for human emotion.
In this Profile, I summarize that journey, discuss why the future of emotion science hinges on big data and artificial intelligence, and provide resources that I hope will make it easier for scientists without a computational background to begin applying state-of-the-art AI to their data.
The Need for “Steel Manning” in Emotion Science
Before delving into big data and AI, let us return to the question of why emotion science has not reached much consensus.
In philosophy, there’s a practice called “steel manning”—before critiquing an argument, one restates it in a manner with which its proponents would agree. It’s the opposite of “straw manning.” I believe this is something that emotion scientists need to start doing more often; if they did, I think the need for bigger data and new methods would be more apparent.
Proponents of one popular theory of emotion often begin papers by pointing out the misconception that emotions are completely variable across cultures. Their evidence to the contrary shows consistency in emotional behavior—across individuals, demographics, cultures, contexts, and so forth.
Proponents of the contrasting theory often point out that there are scientists who believe a fixed number of emotions are universal. Their evidence shows variability in emotional behavior—again, across individuals, demographics, cultures, contexts, etc.
Papers from each approach begin with the premise that some people think there is only consistency, or only variability, in a set of emotional behaviors. But to “steel man” these theories, you would have to start by acknowledging that everyone already agrees that there is both consistency and variability in emotional behavior. The real question is, what exactly is consistent in emotional behavior? What is it that varies across individuals, demographics, cultures, and contexts?
If the goal is to explain human behavior, then this is where the meat of emotion theory lies. It is not in broadly writ notions of universality or variability in human behavior across cultures. It is in the more specific, auxiliary claims that you often find closer to the results section of a paper, such as the following:
- People in different cultures perceive similar levels of “valence”—the level of pleasure or displeasure—in a facial expression. They also perceive similar levels of “arousal”—the level of calmness or excitement. Other dimensions, like “amusement,” are variable across cultures, except insofar as they are correlated with valence and arousal [2–4].
- People in different cultures perceive similar levels of emotions like “amusement” in facial expressions, above and beyond similarities in valence and arousal [5].
- People in different cultures perceive things like unexpectedness, abruptness, and goal-congruence in facial expressions, and this is what explains the perception of emotions like “amusement” [6]
These are “steel man” versions of claims that have been made in prominent papers in emotion science. They are testable claims about what it is precisely that is consistent across people and what it is that is variable. They are mutually exclusive. If we are to take these claims seriously, we must ask next: What does it really take to put them to the test?
A Computational Approach to Emotion
Scientists have come up with dozens of theoretical constructs to explain emotional behaviors: from the “basic six” emotions—anger, disgust, fear, happiness, sadness, and surprise – to valence and arousal, to dimensions like unexpectedness, abruptness, and goal-congruence. In everyday life, people commonly use dozens of different concepts to describe their emotions. Meanwhile, emotional behaviors have dozens, if not hundreds, of parameters—40 or so facial muscles, lots of ways we can move our bodies, lots of ways we can manipulate our voice, and so forth. And we experience emotion in an incredibly wide variety of situations.
What this means is that to really put any of the claims I laid out above to the test, you need to examine a plethora of theoretical constructs, everyday concepts, emotional behaviors, or experiences, and map out their relationships. To do this systematically is to derive what I have termed a “semantic space” of emotion.
Semantic spaces of emotion are defined by three properties (Figure 1A). The first is their dimensionality: How many different kinds of emotion are there? The second is the distribution of states within the space: Are there discrete boundaries between emotions, or is there overlap [7,8]? The third is the conceptualization of emotion: What concepts most precisely capture the variation in the emotional experiences and emotional expressions that people consider to be distinct [9,10]? Do experiences and expressions correspond to specific emotions, like “interest,” “sadness,” and amusement,” or broader evaluations like “valence” and “arousal” [2,7,11] or “certainty” [12]?
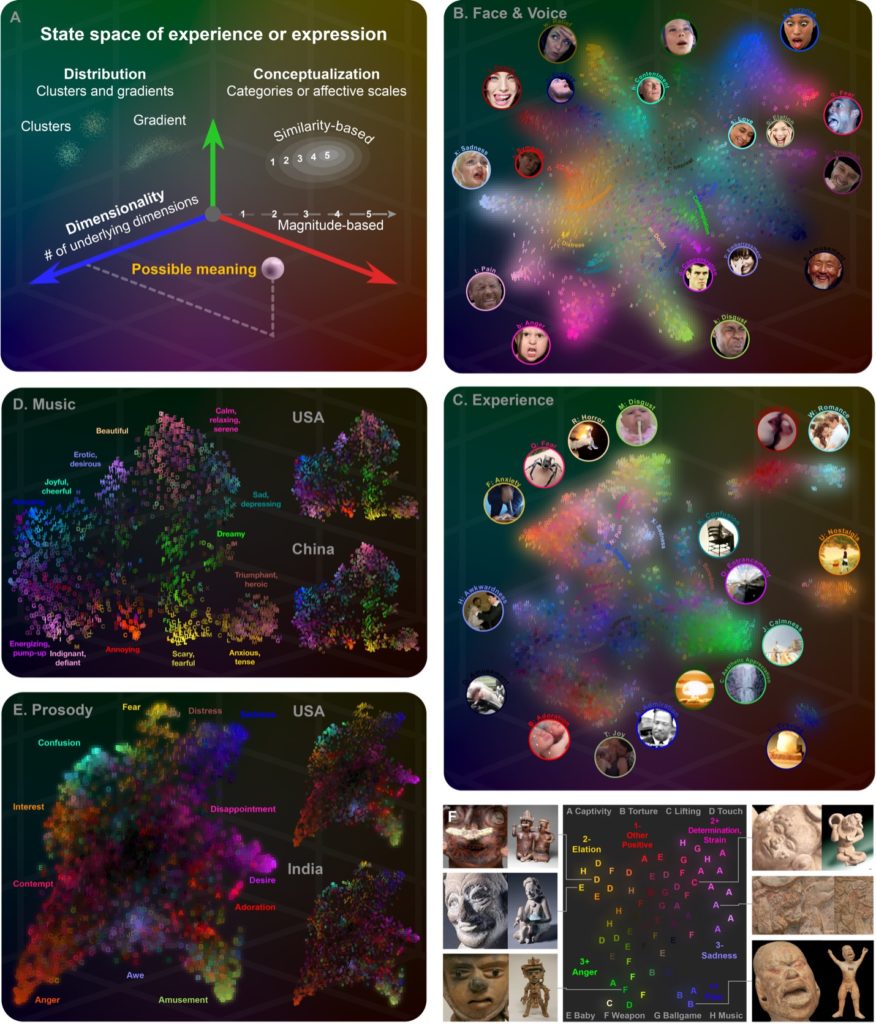
To capture semantic spaces of emotion, we needed new kinds of data and new statistical approaches. It turns out that the small number of emotions and prototypical stimuli [3,13] that are by far the most studied capture only a fraction, about 30%, of the information conveyed by emotion concepts and emotional expressions [14]. Accurately characterizing the meaning of what we say we feel and what we express turns out to require measuring participants’ responses to vast arrays of evocative stimuli and expressions [15]. It requires moving beyond traditional statistical approaches like recognition accuracy [13] and factor analysis [2,12], approaches that presuppose either one-to-one mappings between emotional behaviors and concepts (e.g., “anger”) or that these relationships reduce to a small number of dimensions.
Semantic spaces of emotion satisfy a broader goal: to separate signal—what the data at hand is capable of explaining—from noise. To carry signal, or meaning about emotion, all instances of a particular behavior (e.g., a smile) do not need to map to the same emotional state. Indeed, our studies have shown that the same facial expressions used in everyday life are sometimes used in multiple, very distinct contexts, such as sentimental expressions of musical performers that resemble expressions of pain [16].
Grounded in these principles, we have used large-scale data and new statistical tools to derive semantic spaces of emotion in facial-bodily expression [18], nonverbal vocalization [19], speech prosody [21], and the feelings evoked by music [20] and video [8], within and across cultures [20,21]. In different studies, we had thousands of people evaluate music samples provided by hundreds of other participants in the U.S. and China; speech samples recorded by hundreds of actors in five countries; vocalizations from improv actors; and much more (Figure 1B-E). We even toured museums to study facial expressions in ancient American sculptures (Figure 1F). Our results were both surprising and consistent. Over 25 emotions are associated with distinct profiles of behavior, many more than are typically account for in studies of emotion. Specific emotions like “amusement,” more than valence and arousal, organize experience, expression, and neural processing. Emotions are not discrete, but systematically blended.
When we move beyond traditional models to study these broader semantic spaces, we uncover much more depth and nuance in human emotion than emotion scientists are used to accounting for. Many of these findings harken back to the observations of Charles Darwin, who described similarities and differences in dozens of emotional behaviors across mammalian species and diverse cultures [23].
Advancing Emotion Science with Artificial Intelligence
The goal of emotion science is not just to characterize emotional behavior. It’s to understand how these behaviors shape relationships from the first moments of life [24], guide judgment, decision-making, and memory [25,26], and contribute to our health [27] and well-being [28]. To better understand these processes, it is critical to examine how emotional behavior unfolds in everyday life around the world.
Evidence of this kind is surprisingly lacking in emotion science. It is extremely difficult to capture expressive behavior in real-life contexts that trigger strong emotions. The hand-coding of emotional expressions is time consuming [29]. Moreover, because emotional expressions and the contexts in which they occur are complex, estimating associations between them requires extensive data [30]. For scientists to study emotional behavior in real life at such a scale, we needed new methods.
A few years into grad school, I was approached by tech companies attempting to build AI with empathy who were drawn to my research on semantic spaces of emotion. People were interested in building defenses against bullying, harassment, and depression into social media apps; methods to detect frustration and tiredness into voice assistants; tools to diagnose mental health conditions; and more.
One of the companies I worked with was Google, where I helped establish research efforts focused on the recognition of emotional behaviors. Over a couple of years, I helped develop the most accurate and nuanced algorithm ever built for measuring facial expression. This was a deep neural network (DNN), an algorithm that applied multiple layers of transformation to videos to predict the emotions perceived in facial expressions. My involvement in this effort created an incredible opportunity to study, for the first time, how facial expressions systematically co-vary with specific social contexts around the world.
In our first study using our DNN, we examined emotional behavior at a scale previously unheard of in emotion science: 16 types of facial expression in thousands of contexts found in 6 million videos from 144 countries [16]. We found that each kind of facial expression had distinct associations with a set of contexts that were 70% preserved across 12 world regions. Consistent with these associations, regions varied in how frequently different facial expressions were produced as a function of which contexts were most salient. These results revealed fine-grained patterns in human facial expressions that were well-preserved across the modern world.
In a second study conducted primarily at the University of California, Berkeley, we recorded 45,231 reactions to 2,185 evocative videos (Figure 2), largely in North America, Europe, and Japan, collecting participants’ self-reported experiences in English or Japanese and DNN annotations of facial movement [31]. Facial expressions predicted at least 12 dimensions of experience, despite individual variability. We also identify culture-specific display tendencies—many facial movements differed in intensity in Japan compared to the U.S. and Europe, but represented similar experiences. With newfound precision, these results revealed how people experience and express emotion around the world: in high-dimensional, categorical, and complex fashion.
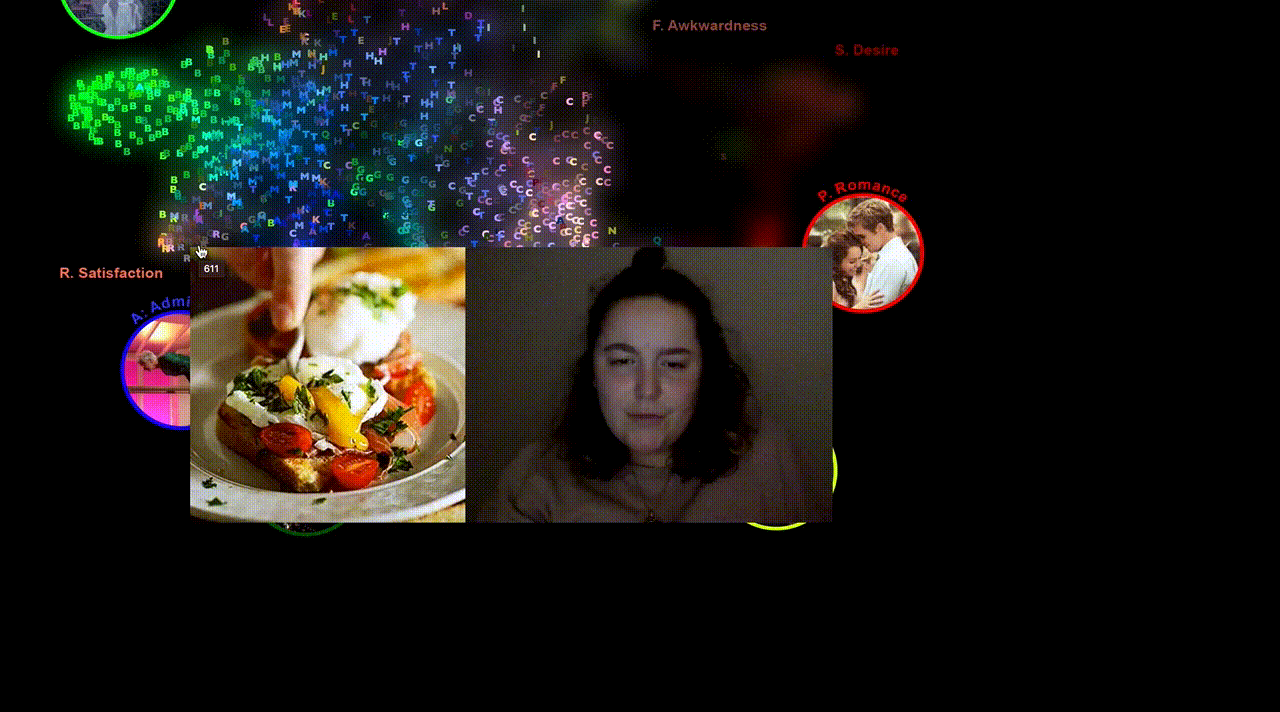
The Future of Big Data and AI in Emotion Science
Unfortunately, there were still important limitations in AI for emotion recognition. The algorithms that had been trained to date, including our DNN, were not well suited to recognize emotional behaviors found more rarely in publicly available data, such as expressions of disgust or fear. They were also confounded by certain perceptual biases—for instance, our DNN labeled anyone wearing sunglasses as expressing pride (as a result, we threw away many of its outputs). Finally, and perhaps most importantly, the DNN trained at Google wasn’t publicly available for use by other researchers.
To train more accurate algorithms, we would need large-scale, globally diverse data with a multitude of emotional expressions and contexts. To advance the field of emotion science, we would also need to clear the way to share these AI tools with other researchers.
These are the goals of the private lab I started seven months ago, Hume AI (hume.ai). Hume has been gathering a new kind of rich, globally diverse, psychologically valid emotion data at scale. We have now gathered 3 million self-report and perceptual judgments of 1.5 million human emotional behaviors (Figure 3). With this data, we have trained algorithms that can infer human emotional behavior in the face (https://hume.ai/solutions/facial-expression-model) and voice (https://hume.ai/solutions/vocal-expression-model) with more accuracy and nuance than ever before.
(We are now making our algorithms available free of charge to research groups with compelling data to analyze. If you are interested in using our AI algorithms in your research, please feel free to reach out at hello@hume.ai.)
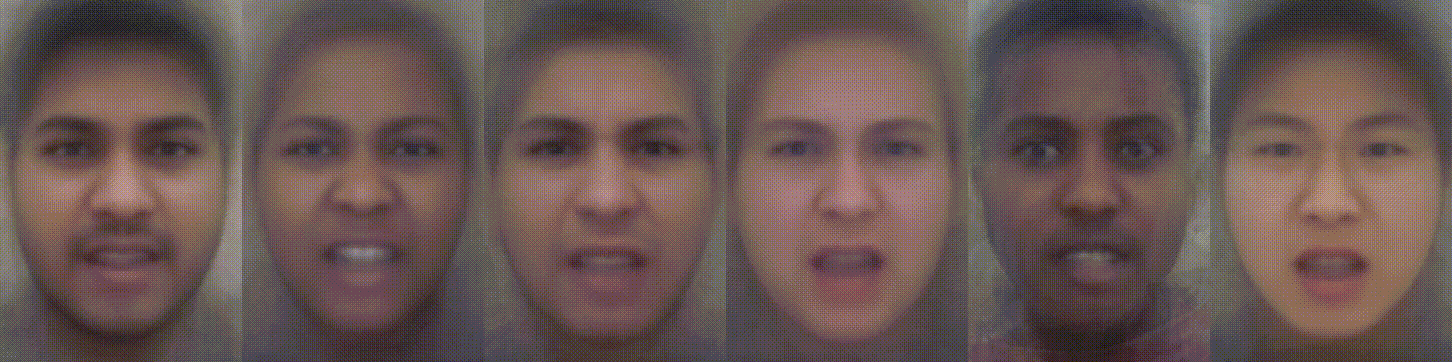
The Way Forward
With new data and methods, I hope that researchers take the opportunity to look beyond entrenched theories and debates. Rather than look to defend broadly writ notions of universality or variability in human behavior, we can ask, with newfound precision, what exactly is consistent in emotional behavior? What is it that varies across individuals, demographics, cultures, and contexts? What do emotional behaviors, whether universal or culture-specific, indicate about our present beliefs, feelings, and relationships?
I hope that the field of emotion science looks beyond our disagreements, real or imagined—particularly disagreements in terminology, emphasis, or in the questions we feel are most important to answer—toward advances in the substance of our understanding of human emotional behavior. Such advances should build on the fundamental consensus we all share, in and out of academia, that emotional behavior is not meaningless, nor is its meaning straightforward. That a human being is justified in reacting differently to a laugh coming from their living room than to a blood-curdling scream, even if they cannot be sure what it indicates. To draw quantitative insights that do justice to the nuances of human emotion, we will have to come to terms with the fact that what has impended progress in the field is not so much a deficiency in theory, but a deficiency in the tools scientists have had at their disposal to measure emotion behaviors with sufficient precision and at a sufficient scale to draw useful inferences from their occurrence in everyday life.
References
1 Hume, D. (2003) A treatise of human nature, Courier Corporation.
2 Russell, J.A. (2003) Core Affect and the Psychological Construction of Emotion. Psychol. Rev. 110, 145–172
3 Barrett, L.F. et al. (2019) Emotional Expressions Reconsidered: Challenges to Inferring Emotion From Human Facial Movements. Psychol. Sci. Public Interes. 20, 1–68
4 Gendron, M. et al. (2015) Cultural Variation in Emotion Perception Is Real: A Response to Sauter, Eisner, Ekman, and Scott (2015). Psychol. Sci. 26, 357–359
5 Sauter, D.A. et al. (2015) Emotional Vocalizations Are Recognized Across Cultures Regardless of the Valence of Distractors. Psychol. Sci. 26, 354–356
6 Gentsch, K. et al. (2015) Appraisals generate specific configurations of facial muscle movements in a gambling task: Evidence for the component process model of emotion. PLoS One 10,
7 Barrett, L.F. (2006) Are Emotions Natural Kinds? Perspect. Psychol. Sci. 1, 28–58
8 Cowen, A.S. and Keltner, D. (2017) Self-report captures 27 distinct categories of emotion bridged by continuous gradients. Proc. Natl. Acad. Sci. U. S. A. 114, E7900–E7909
9 Shaver, P. et al. (1987) Emotion Knowledge: Further Exploration of a Prototype Approach. J. Pers. Soc. Psychol. 52, 1061–1086
10 Scherer, K.R. and Wallbott, H.G. (1994) Evidence for Universality and Cultural Variation of Differential Emotion Response Patterning. J. Pers. Soc. Psychol. 66, 310–328
11 Barrett, L.F. (2006) Valence is a basic building block of emotional life. J. Res. Pers. 40, 35–55
12 Smith, C.A. and Ellsworth, P.C. (1985) Patterns of Cognitive Appraisal in Emotion. J. Pers. Soc. Psychol. 48, 813–838
13 Elfenbein, H.A. and Ambady, N. (2002) On the universality and cultural specificity of emotion recognition: A meta-analysis. Psychol. Bull. 128, 203–235
14 Cowen, A.S. et al. (2019) Mapping the Passions: Moving from Impoverished Models to a High Dimensional Taxonomy of Emotion. Psychol. Sci. Public Interes. in press,
15 Cowen, A.S. et al. (2019) Mapping the Passions: Toward a High-Dimensional Taxonomy of Emotional Experience and Expression. Psychol. Sci. Public Interes. 20, 69–90
16 Cowen, A.S. et al. Sixteen facial expressions occur in similar contexts worldwide. Nature
17 Cowen, A.S. and Keltner, D. (2021) Semantic space theory: A computational approach to emotion. Trends Cogn. Sci.
18 Cowen, A.S. and Keltner, D. (2019) What the Face Displays: Mapping 28 Emotions Conveyed by Naturalistic Expression. Am. Psychol. DOI: 10.1037/amp0000488
19 Cowen, A.S. et al. (2018) Mapping 24 Emotions Conveyed by Brief Human Vocalization. Am. Psychol. 22, 274–276
20 Cowen, A.S. et al. (2020) What music makes us feel: Uncovering 13 kinds of emotion evoked by music across cultures. Proc. Natl. Acad. Sci.
21 Cowen, A.S. et al. (2019) The primacy of categories in the recognition of 12 emotions in speech prosody across two cultures. Nat. Hum. Behav. 3, 369–382
22 Cowen, A.S. and Keltner, D. (2020) Universal emotional expressions uncovered in art of the ancient Americas: A computational approach. Sci. Adv.
23 Darwin, C. and Darwin, F. (2009) The expression of the emotions in man and animals, Oxford University Press.
24 Niedenthal, P.M. and Ric, F. (2017) Expression of Emotion. In Psychology of Emotion (2nd edn) (L. F. Barrett, M. Lewis, & J. M. H. J., ed), pp. 98–123, Guilford Press
25 Keltner, D. et al. (2014) The Sociocultural Appraisals, Values, and Emotions (SAVE) Framework of Prosociality: Core Processes from Gene to Meme. Annu. Rev. Psychol. 65, 425–460
26 Holland, A.C. and Kensinger, E.A. Emotion and autobiographical memory. , Physics of Life Reviews, 7. (2010) , 88–131
27 Kok, B.E. et al. (2013) How Positive Emotions Build Physical Health: Perceived Positive Social Connections Account for the Upward Spiral Between Positive Emotions and Vagal Tone. Psychol. Sci. 24, 1123–1132
28 Diener, E. et al. The evolving concept of subjective well-being: the multifaceted nature of happiness. Advances in Cell Aging and Gerontology, 15. (2003) , 187–219
29 Cohn, J.F. et al. (2007) Observer-based measurement of facial expression with the Facial Action Coding System. In The handbook of emotion elicitation and assessment (Coan, J. A. and Allen, J. J. B., eds), pp. 203–221, Oxford University Press
30 Gatsonis, C. and Sampson, A.R. (1989) Multiple Correlation: Exact Power and Sample Size Calculations. Psychol. Bull. 106, 516–524
31 Cowen, A.S. et al. How emotion is experienced and expressed in multiple cultures: A large-scale experiment. under Rev. at <https://psyarxiv.com/gbqtc>