Rachael Jack, Institute of Neuroscience and Psychology, University of Glasgow
Our Aim & Approach
August 2015 – In our research group, we aim to understand social communication – i.e., how humans transmit and decode information to support social interactions. Our primary current focus is on emotion communication by means of facial expressions. To shed light on this, we use a multidisciplinary approach that combines vision science and psychophysics, cultural psychology, cognitive science, and 4D computer graphics. Here, I will highlight the strengths and broad potential of our approach and consider future directions. First, it is useful to step back and briefly consider what social communication is.
Social Communication as a System of Information Transmission and Decoding
Social communication is much like many other communication systems (e.g., Morse coding, pheromones, mating calls, bacterial quorum sensing) – a dynamic process of transmitting and decoding information.

Communication is the act of sending information that affects the behavior of another individual (e.g., Dukas 1998, Shannon 2001, Scott-Phillips 2008). Although the specifics of defining communication are widely discussed (e.g., whether sender and/or receiver should benefit from the exchange), our focus is on understanding the transmission and decoding of information as shown in Figure 1. Briefly stated, the sender encodes a message (“I feel angry”) into an information bearer (e.g., a facial expression) and transmits it to a receiver (e.g., a human visual system). The receiver must first detect then decode the transmitted information using prior knowledge (i.e., mental representations) to derive a meaning (e.g., see Schyns 1998). For example, if the wrinkled nose and bared teeth in a face corresponds with the receiver’s prior knowledge of ‘anger,’ he/she will perceive that “he/she feels angry.” Therefore, understanding any system of communication requires identifying which transmitted information reliably elicits a particular perception in the receiver.
The Face is a Highly Complex Source of Social Information
One of the most powerful tools in social communication is the face (but see also voice and bodies, e.g., Belin, Fillion-Bilodeau et al. 2008, de Gelder 2009, Sauter, Eisner et al. 2010). As illustrated in Michael Jackson’s Black or White music video below, the stream of changing faces vividly elicits a sequence of inferences based on the face – e.g., identity (e.g., Gauthier, Tarr et al. 1999), gender/sex (e.g., Little, Jones et al. 2008), age (e.g., Van Rijsbergen, Jaworska et al. 2014), race/ethnicity (e.g., Tanaka, Kiefer et al. 2004), sexual orientation (e.g., Rule and Ambady 2008), health (e.g., Grammer and Thornhill 1994), attractiveness (e.g., Perrett, Lee et al. 1998), emotions (e.g., Ekman 1972), personality traits (e.g., Willis and Todorov 2006), pleasure (e.g., Fernández-Dols, Carrera et al. 2011), pain (e.g., Williams 2002), social status (e.g., in pigmentocracies where skin tone determines social status, see Telles 2014), and deception (e.g., Krumhuber and Kappas 2005).
As a transmitter of information about multiple and complex social categories, the face represents a high dimensional dynamic information space (see also Fernandez-Dols 2013). To illustrate, the face has numerous independent muscles (Drake, Vogl et al. 2010), which can be combined to produce intricate dynamic patterns (i.e., facial expressions). Variations in facial morphology (i.e., shape and structure), color (e.g., pigmentation, see Pathak, Jimbow et al. 1976), texture (e.g., wrinkles, scarring), and facial adornments (e.g., cosmetics/painting, tattooing, piercings/jewelry, hair) each provide further rich sources of variance (see Dimensions of Face Variance Movie below). Therefore, identifying which segments of the high dimensional information space subtends different social judgments remains a major empirical challenge. How can we tackle this successfully?
Broadening Theoretical and Empirical Approaches to Social Communication
To address the challenge of extracting socially relevant information from the face, traditional approaches primarily use theoretical knowledge and naturalistic observations to select and test small sections of the high dimensional information space. Most notably, based on Darwin’s groundbreaking theory of the biological origins of facial expressions (Darwin 1999/1872), Ekman’s pioneering work proposed that a specific set of facial expressions, each characterized by a pattern of individual face movements called Action Units (AUs, see Ekman and Friesen 1978 for all AU patterns), communicates six basic emotions (‘happy,’ ‘surprise,’ ‘fear,’ ‘disgust,’ ‘anger’ and ‘sad’) across all cultures. In several cross-cultural recognition studies (e.g., Ekman, Friesen et al. 1987, Matsumoto and Ekman 1989, Biehl, Matsumoto et al. 1997), these specific AU patterns elicited above chance recognition performance (e.g., >16.7 % accuracy in a standard 6-alternative forced choice task, i.e., 1/6 = chance) across different cultures. Consequently, these AU patterns became widely acknowledged as universal (see Izard 1994 for a review) and the gold standard in research across broad fields.
These classic works remain undoubtedly influential, inspiring an era of cross-cultural recognition studies that have dominated the field for over 40 years. However, such an approach casts a relatively narrow light on understanding which facial expressions are universal and which are culture-specific (see also Russell 1994, Elfenbein and Ambady 2002, Jack 2013, Nelson and Russell 2013). Specifically, using chance performance (i.e., >16.7% accuracy in a standard 6-alternative forced choice task) as a minimal threshold criterion to demonstrate universality potentially masks significant cultural differences in recognition accuracy, because any variance in performance would be considered as equally universal. Furthermore, most recognition studies have focused on a limited (and limiting) set of basic emotions, neglecting many other emotion categories in the process (e.g., delighted, anxious). Consequently, knowledge has remained restricted to a small set of static AU patterns that communicate only six emotions primarily in Western culture, disregarding a large proportion of the human population (Henrich, Heine et al. 2010).
Identifying the dynamic AU patterns that communicate different emotions and other socially relevant categories (e.g., personality traits, other mental states etc.) requires considering the full high dimensional information space of possible face movements. Here, we present such an approach.
Probing the ‘Receptive Fields’ of Social Perception Using Psychophysics
To identify which specific patterns of facial movements – that is, segments of the high dimensional dynamic information space – communicate emotions in a given culture, we use an approach analogous to probing the receptive fields of sensory neurons. For example, Hubel and Wiesel identified which visual information V1 cells in the primary visual cortex code by presenting variations of simple black bars differing in orientation, length, direction of movement and so on, and measuring which neurons fire when presented with specific stimulus features (Hubel and Wiesel 1959). Similarly, we probe the ‘receptive fields’ of social perception by presenting specific dynamic AU patterns and measuring the receiver’s perceptual categorization responses. In other words, we use the receiver much like a metal detector to identify, in the high dimensional information space, the specific dynamic AU patterns associated with a given perceptual category (e.g., ‘happy’) in a given culture. Such an approach is fundamental to psychophysics – a field that aims to measure the relationship between objectively measurable information in the external environment (i.e., physical stimuli such as dynamic AU patterns) and its interpretation by an observer (i.e., subjective perception).
To illustrate, suppose that we aim to identify the facial movements that communicate the six classic emotions – ‘happy,’ ‘surprise,’ ‘fear,’ ‘disgust,’ ‘anger’ and ‘sad’ – in an unknown culture such as the Sentinelese people of the Andaman Islands situated in the Bay of Bengal. With no a priori knowledge of these facial expressions, we could select AU patterns agnostically – i.e., create random facial expressions – and ask members of that culture to select those that accurately represent each of the six emotions. Figure 2 illustrates our method using an example trial.
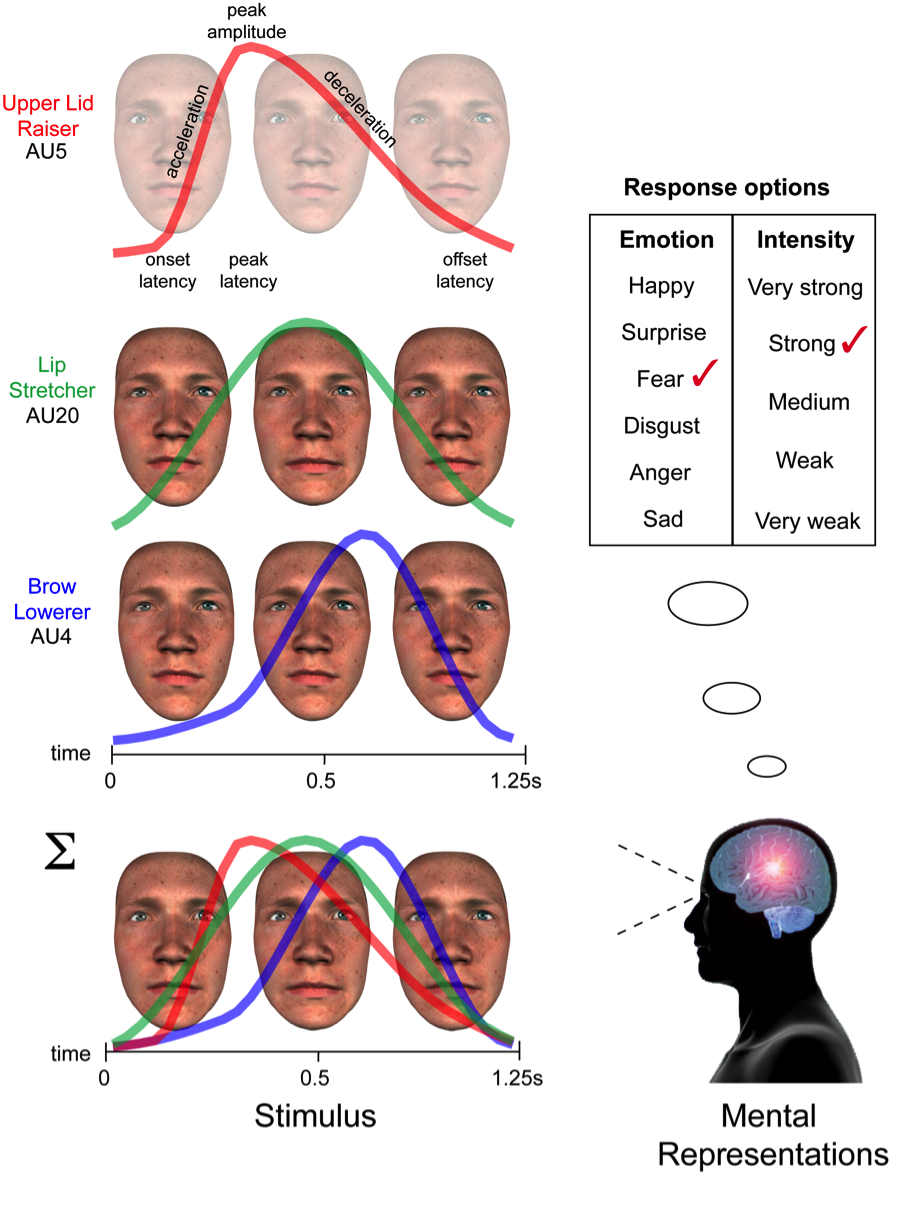
On each experimental trial, a dynamic facial expression computer graphics platform – the Generative Face Grammar (GFG, Yu, Garrod et al. 2012) – randomly samples a subset of AUs from a core set of 42 AUs (e.g., in Figure 2 three AUs are selected: Upper Lid Raiser – AU5 color-coded in red, Lip Stretcher – AU20 in green, and Brow Lowerer – AU4 in blue). The GFG then ascribes a random movement to each AU by selecting random values for each of six temporal parameters – onset latency, acceleration, peak latency, peak amplitude, deceleration and offset latency (see illustration in top row, and color-coded curves representing each AUs activation over time). The dynamic AUs are then combined to produce a photo-realistic random facial movement, illustrated here with three snapshots across time (see also Stimulus Generation movie below).
The naïve receiver from the Sentinelese culture categorizes the stimulus according to one of the six classic emotions and rates the intensity of the emotion perceived (here, ‘fear of strong intensity’) if the random face movements form a pattern that correlates with their mental representation (i.e., perceptual expectation) of that facial expression. Otherwise, the receiver selects ‘other’ if none of the response options accurately describe the stimulus. Over many such trials, we therefore obtain a measure of the relationship between segments of the high dimensional information space (i.e., dynamic AU patterns) and the receiver’s perceptual categorization response (i.e., the six classic emotions at different levels of intensity). Statistical analyses of these relationships using a method called ‘reverse correlation’ (Ahumada and Lovell 1971) can reveal the specific dynamic facial expression patterns that reliably communicate these emotions in this culture. Thus, we can derive the psychophysical laws of subjective high-level constructs (e.g., emotion categories) as they relate to the objectively measurable physical aspects of the face.
Doing so in different cultures (e.g., Western and East Asian, different socio-economic classes, or age groups) can then reveal whether dynamic facial expressions are similar or different across cultures, and if so, how. For example, although the six classic facial expressions of emotion have largely been considered universal, differences in recognition accuracy across cultures, and proposed culture-specific accents/dialects (e.g., Labarre 1947, Marsh, Elfenbein et al. 2003, Elfenbein, Beaupre et al. 2007) call into question the universality thesis (see Russell 1994 for reviews, Elfenbein and Ambady 2002). Additionally, empirical knowledge of facial expressions of emotion across cultures is generally limited to static and typically posed displays (e.g., Ekman and Friesen 1975, Ekman and Friesen 1976, Matsumoto and Ekman 1988, Elfenbein, Beaupre et al. 2007) that are recognized primarily in Western culture (e.g., Matsumoto and Ekman 1989, Matsumoto 1992, Moriguchi, Ohnishi et al. 2005, Jack, Blais et al. 2009). Using dynamic stimuli, our method can better characterize the dynamic AU patterns that communicate emotions, or indeed any socially relevant category such as personality traits (e.g., trustworthiness) or cognitive states (e.g., confusion), simply by changing the response options.
Revealing Cultural Specificities in Dynamic Facial Expressions of Emotion
Using this approach, we have identified the specific dynamic AU patterns that communicate the six classic emotions – ‘happy,’ ‘surprise,’ ‘fear,’ ‘disgust,’ ‘anger’ and ‘sad’ – in different cultures (Western and East Asian), revealing three main cultural differences (Jack, Garrod et al. 2012). Figures 3 – 5 show the results.
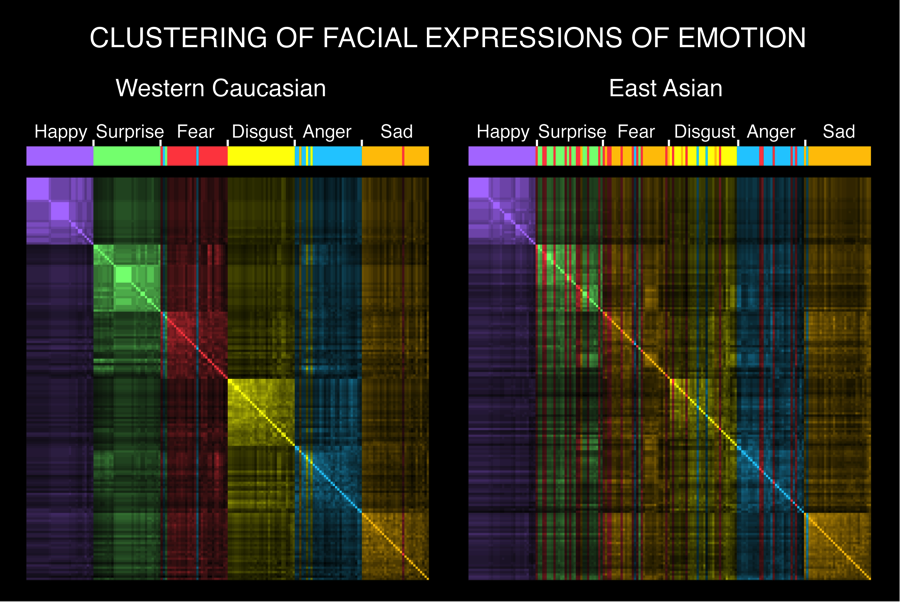
First, as shown in Figure 3, Western facial expressions formed six distinct and emotionally homogeneous clusters. For example, all Western ‘happy’ facial expression AU patterns are more similar to each other (i.e., high within-category similarity) than to other facial expressions of emotions, such as ‘anger’ or ‘sad’ (i.e., low between-category similarity). The uniform coloring of each cluster reflects the emotion category homogeneity of each cluster. In contrast, East Asian facial expression AU patterns showed similarities between different emotion categories, as indicated by the heterogeneous color-coding across ‘surprise,’ ‘fear,’ ‘disgust’ and ‘anger,’ reflecting a culture-specific departure from the view that emotion communication comprises six universally represented categories (e.g., Ekman 1992).
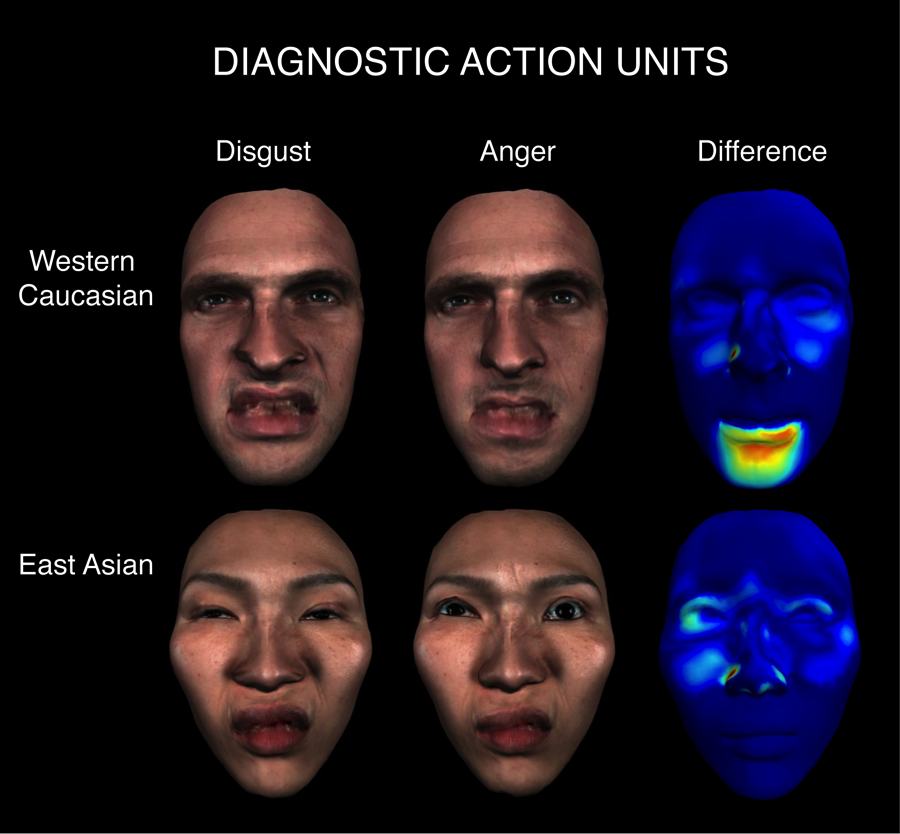
Second, as shown by the color-coded difference face maps in Figure 4, in Western culture facial expressions of ‘disgust’ and ‘anger’ differ according to the mouth, whereas in East Asian culture the eyes differ. For example, note the narrowing of the eyes in ‘disgust’ vs. the widening of the eyes in ‘anger.’
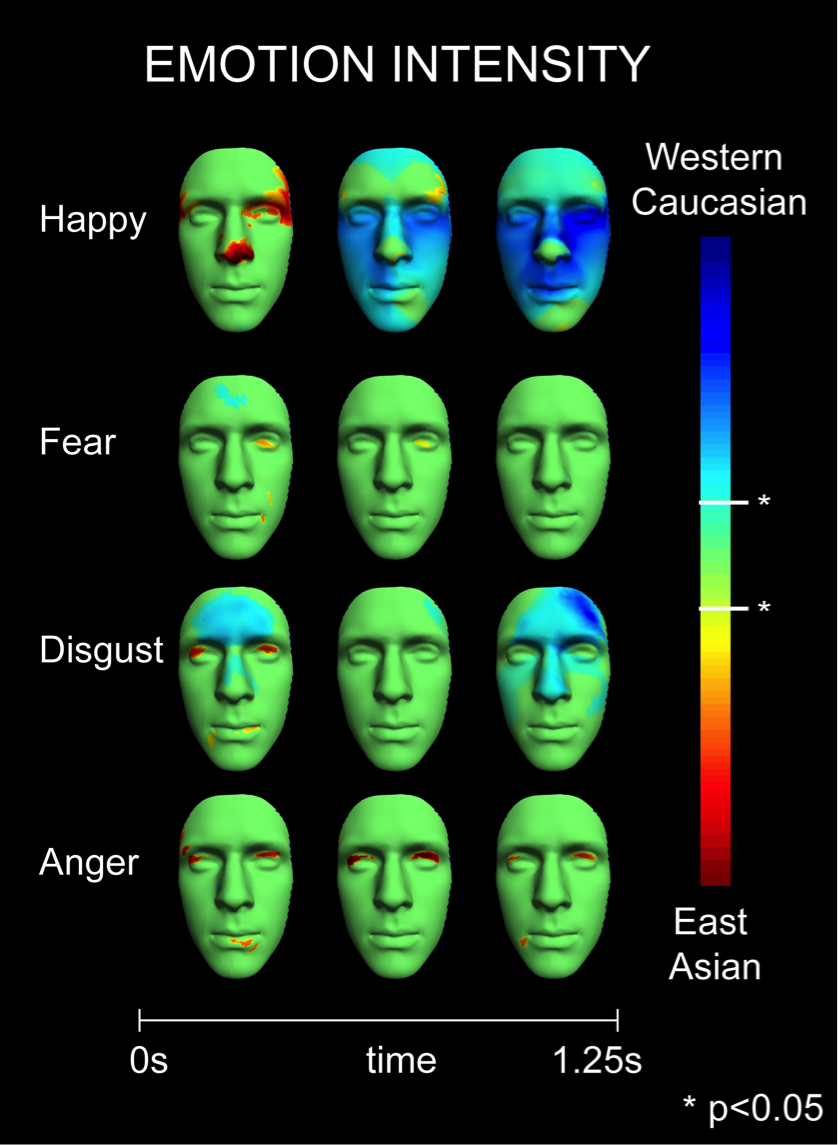
In Figure 5, color-coded face maps – illustrated here with ‘happy,’ ‘fear,’ ‘disgust,’ and ‘anger’ – show cultural differences in the communication of emotional intensity. For example, early red colored areas show that East Asian facial expressions use the eye region to convey emotional intensity – a finding that is mirrored by East Asian emoticons where (^.^) represents happy and (>.<) represents angry (see also Yuki, Maddux et al. 2007). Here, by mapping the relationship between dynamic AU patterns and the perception of the six classic emotions in different cultures, we precisely characterized cultural specificities in facial expressions of emotion, questioning and refining the assumption of universality.
Beyond the Classic Six: Complex Emotions, Personality Traits and Mental States
Of course, our method can be used to identify the dynamic facial expressions that communicate virtually any social category. For example, extending beyond classic emotions, we have used our method to identify, in Western Caucasian and East Asian cultures, dynamic facial expressions of a much broader spectrum of emotions such as ‘pride,’ ‘shame,’ ‘excited,’ ‘amazed,’ ‘anxious,’ and ‘grief’ (Sun, Garrod et al. 2013), providing a richer characterization of emotion communication within and across cultures. Similarly, we have revealed intricacies in the transmission of facial movements over time. Early biologically based face movements such as eye widening and nose wrinkling (Susskind, Lee et al. 2008) are common to both ‘fear’ and ‘surprise,’ and ‘disgust’ and ‘anger,’ respectively, which supports the discrimination of only four emotion categories – ‘happy,’ ‘sad,’ ‘fear’/‘surprise,’ ‘disgust’/‘anger.’ Later in the signalling dynamics, diagnostic face movements such as eyebrow raising or upper lip raising support the discrimination of all six emotion categories (Jack, Garrod et al. 2014). We have also characterized dynamic facial expressions communicating personality traits – attractiveness, trustworthiness and dominance – and shown that such facial expressions, when displayed by specific face morphologies, significantly changes the social perception derived from the face.
For example, the receiver’s social perception of a unexpressive face morphology can transform from highly untrustworthy to highly trustworthy when the face displays a trustworthy facial expression (Gill, Garrod et al. 2014). Finally, by identifying the dynamic facial expressions communicating the mental states of ‘thinking,’ ‘interested,’ ‘bored’ and ‘confused’ in different cultures – a valuable skill during any social exchange – we revealed the precise face information sources that produce cross-cultural miscommunication and support mutual understanding. Specifically, whereas ‘interested’ and ‘bored’ are recognized across cultures due to similar AU patterns, ‘confused’ shows cultural specific AU patterns that significantly impacts cross-cultural communication (Chen in press).
Conclusions and Future Work
Our approach uses psychophysics to agnostically select segments of the high dimensional dynamic information space of the face and measure the receiver’s perceptual social categorization responses. Thus, we derive the psychophysical laws of social perception. The novelty and strength of our approach is that, rather than using subjective assumptions to select and test a small set of AU patterns, we ask individuals from a given culture to select from a wide array which facial expressions accurately communicate different emotions, or other socially relevant categories, in their culture. By using a data-driven approach, we can objectively and precisely characterize how the face – a highly complex source of social information – dynamically communicates a broad range of social categories in any culture. Our next goal is to extend our method to two other relevant dimensions of the face – complexion and morphology – to understand how each individually and in combination contributes to the perception of social categories in different cultures. We anticipate that our data-driven results will contribute to the development of existing theories of social and facial communication, and generate new conceptual advances, thereby both nurturing and benefitting from a symbiotic theory-data relationship.
Acknowledgements
Our research is funded by The Economic and Social Research Council and Medical Research Council (United Kingdom; ESRC/MRC-060-25-0010; ES/K001973/1; ES/K00607X/1), and British Academy (SG113332).
References
Ahumada, A. and J. Lovell (1971). “Stimulus features in signal detection.” Journal of the Acoustical Society of America 49(6, Pt. 2): 1751-1756.
Belin, P., S. Fillion-Bilodeau and F. Gosselin (2008). “The Montreal Affective Voices: a validated set of nonverbal affect bursts for research on auditory affective processing.” Behavior research methods 40(2): 531-539.
Biehl, M., D. Matsumoto, P. Ekman, V. Hearn, K. Heider, T. Kudoh and V. Ton (1997). “Matsumoto and Ekman’s Japanese and Caucasian Facial Expressions of Emotion (JACFEE): Reliability Data and Cross-National Differences.” Journal of Nonverbal Behavior 21(1): 3-21.
Chen, C., Garrod, O.G.B., Schyns, P.G., Jack, R.E. (in press). “The face is the mirror of the cultural mind.” Journal of vision.
Darwin, C. (1999/1872). The Expression of the Emotions in Man and Animals. London, Fontana Press.
de Gelder, B. (2009). “Why bodies? Twelve reasons for including bodily expressions in affective neuroscience.” Philosophical Transactions of the Royal Society B: Biological Sciences 364(1535): 3475-3484.
Drake, R. L., A. Vogl and A. W. Mitchell (2010). Gray’s anatomy for students, Philadelphia, Churchill Livingstone, Elsevier.
Dukas, R. (1998). Cognitive ecology: the evolutionary ecology of information processing and decision making, University of Chicago Press.
Ekman, P. (1972). Universals and cultural differences in facial expressions of emotion. Nebraska Symposium on Motivation. J. Cole, University of Nebraska Press.
Ekman, P. (1992). An argument for basic emotions. Hove, ROYAUME-UNI, Psychology Press.
Ekman, P. and W. Friesen (1978). Facial Action Coding System: A Technique for the Measurement of Facial Movement, Consulting Psychologists Press.
Ekman, P. and W. V. Friesen (1975). Unmasking the face: a guide to recognizing emotions from facial clues. Englewood Cliffs, Prentice-Hall.
Ekman, P. and W. V. Friesen (1976). Pictures of Facial Affect. Palo Alto, CA.
Ekman, P., W. V. Friesen, M. O’Sullivan, A. Chan, I. Diacoyanni-Tarlatzis, K. Heider, R. Krause, W. A. LeCompte, T. Pitcairn, P. E. Ricci-Bitti and et al. (1987). “Universals and cultural differences in the judgments of facial expressions of emotion.” Journal of Personality and Social Psychology 53(4): 712-717.
Elfenbein and N. Ambady (2002). “Is there an in-group advantage in emotion recognition?” Psychological Bulletin 128(2): 243-249.
Elfenbein, H. A. and N. Ambady (2002). “On the universality and cultural specificity of emotion recognition: A meta-analysis.” Psychological Bulletin 128(2): 203-235.
Elfenbein, H. A., M. Beaupre, M. Levesque and U. Hess (2007). “Toward a dialect theory: cultural differences in the expression and recognition of posed facial expressions.” Emotion 7(1): 131-146.
Fernández-Dols, J.-M., P. Carrera and C. Crivelli (2011). “Facial behavior while experiencing sexual excitement.” Journal of Nonverbal Behavior 35(1): 63-71.
Fernandez-Dols, J. M. (2013). “Advances in the Study of Facial Expression: An Introduction to the Special Section.” Emotion Review 5(1): 3-7.
Gauthier, I., M. J. Tarr, A. W. Anderson, P. Skudlarski and J. C. Gore (1999). “Activation of the middle fusiform ‘face area’ increases with expertise in recognizing novel objects.” Nat Neurosci 2(6): 568-573.
Gill, D., O. G. Garrod, R. E. Jack and P. G. Schyns (2014). “Facial movements strategically camouflage involuntary social signals of face morphology.” Psychol Sci 25(5): 1079-1086.
Gill, D., O. G. Garrod, R. E. Jack and P. G. Schyns (2014). “Facial movements strategically camouflage involuntary social signals of face morphology.” Psychological science 25(5): 1079-1086.
Grammer, K. and R. Thornhill (1994). “Human ( Homo sapiens ) facial attractiveness and sexual selection: The role of symmetry and averageness.” Journal of Comparative Psychology 108(3): 233-242.
Henrich, J., S. Heine and A. Norenzayan (2010). “The weirdest people in the world?” Behavioral and Brain Sciences 33(2-3): 61-83.
Hubel, D. and T. N. Wiesel (1959). “Receptive fields of single neurons in the cat’s striate visual cortex.” J. Phys 148: 574-591.
Izard, C. E. (1994). “Innate and universal facial expressions: evidence from developmental and cross-cultural research.” Psychol Bull 115(2): 288-299.
Jack, R. E. (2013). “Culture and facial expressions of emotion.” Visual Cognition(ahead-of-print): 1-39.
Jack, R. E., C. Blais, C. Scheepers, P. G. Schyns and R. Caldara (2009). “Cultural Confusions Show that Facial Expressions Are Not Universal.” Current Biology 19(18): 1543-1548.
Jack, R. E., O. G. Garrod and P. G. Schyns (2014). “Dynamic Facial Expressions of Emotion Transmit an Evolving Hierarchy of Signals over Time.” Curr Biol 24(2): 187-192.
Jack, R. E., O. G. Garrod, H. Yu, R. Caldara and P. G. Schyns (2012). “Facial expressions of emotion are not culturally universal.” Proc Natl Acad Sci U S A 109(19): 7241-7244.
Jack, R. E. and P. G. Schyns (2015). “The Human Face as a Dynamic Tool for Social Communication.” Current Biology 25(14): R621-R634.
Krumhuber, E. and A. Kappas (2005). “Moving Smiles: The Role of Dynamic Components for the Perception of the Genuineness of Smiles.” Journal of Nonverbal Behavior 29(1): 3-24.
Labarre, W. (1947). “THE CULTURAL BASIS OF EMOTIONS AND GESTURES.” Journal of Personality 16(1): 49.
Little, A. C., B. C. Jones, C. Waitt, B. P. Tiddeman, D. R. Feinberg, D. I. Perrett, C. L. Apicella and F. W. Marlowe (2008). “Symmetry is related to sexual dimorphism in faces: data across culture and species.” PLoS One 3(5): e2106.
Marsh, A. A., H. A. Elfenbein and N. Ambady (2003). “Nonverbal “accents”: cultural differences in facial expressions of emotion.” Psychological Science 14(4): 373-376.
Matsumoto, D. (1992). “American-Japanese Cultural Differences in the Recognition of Universal Facial Expressions.” Journal of Cross-Cultural Psychology 23(1): 72-84.
Matsumoto, D. and P. Ekman (1988). Japanese and Caucasian Facial Expressions of Emotion (JACFEE) and Neutral Faces (JACNeuF). [Slides]: Dr. Paul Ekman, Department of Psychiatry, University of California, San Francisco, 401 Parnassus, San Francisco, CA 94143-0984.
Matsumoto, D. and P. Ekman (1989). “American-Japanese cultural differences in intensity ratings of facial expressions of emotion.” Motivation and Emotion 13(2): 143-157.
Moriguchi, Y., T. Ohnishi, T. Kawachi, T. Mori, M. Hirakata, M. Yamada, H. Matsuda and G. Komaki (2005). “Specific brain activation in Japanese and Caucasian people to fearful faces.” Neuroreport 16(2): 133-136.
Nelson, N. L. and J. A. Russell (2013). “Universality Revisited.” Emotion Review 5(1): 8-15.
Pathak, M. A., K. Jimbow, G. Szabo and T. B. Fitzpatrick (1976). Sunlight and melanin pigmentation. Photochemical and photobiological reviews, Springer: 211-239.
Perrett, D. I., K. J. Lee, I. Penton-Voak, D. Rowland, S. Yoshikawa, D. M. Burt, S. P. Henzi, D. L. Castles and S. Akamatsu (1998). “Effects of sexual dimorphism on facial attractiveness [see comments].” Nature 394(6696): 884-887.
Rule, N. O. and N. Ambady (2008). “Brief exposures: Male sexual orientation is accurately perceived at 50ms.” Journal of Experimental Social Psychology 44(4): 1100-1105.
Russell, J. A. (1994). “Is there universal recognition of emotion from facial expression? A review of the cross-cultural studies.” Psychological Bulletin 115(1): 102-141.
Sauter, D. A., F. Eisner, P. Ekman and S. K. Scott (2010). “Cross-cultural recognition of basic emotions through nonverbal emotional vocalizations.” Proc Natl Acad Sci U S A 107(6): 2408-2412.
Schyns, P. G. (1998). “Diagnostic recognition: task constraints, object information, and their interactions.” Cognition 67(1-2): 147-179.
Scott-Phillips, T. C. (2008). “Defining biological communication.” J Evol Biol 21(2): 387-395.
Shannon, C. E. (2001). “A mathematical theory of communication.” SIGMOBILE Mob. Comput. Commun. Rev. 5(1): 3-55.
Sun, W., O. G. B. Garrod, P. G. Schyns and R. E. Jack (2013). Dynamic mental models of culture-specific emotions. Vision Sciences Society, Florida, USA.
Susskind, J. M., D. H. Lee, A. Cusi, R. Feiman, W. Grabski and A. K. Anderson (2008). “Expressing fear enhances sensory acquisition.” Nat Neurosci 11(7): 843-850.
Tanaka, J. W., M. Kiefer and C. M. Bukach (2004). “A holistic account of the own-race effect in face recognition: evidence from a cross-cultural study.” Cognition 93(1): B1-9.
Telles, E. (2014). Pigmentocracies: Ethnicity, Race, and Color in Latin America, UNC Press Books.
Van Rijsbergen, N., K. Jaworska, G. A. Rousselet and P. G. Schyns (2014). “With Age Comes Representational Wisdom in Social Signals.” Current Biology.
Williams, A. C. d. C. (2002). “Facial expression of pain, empathy, evolution, and social learning.” Behavioral and brain sciences 25(04): 475-480.
Willis, J. and A. Todorov (2006). “First impressions: making up your mind after a 100-ms exposure to a face.” Psychol Sci 17(7): 592-598.
Yu, H., O. G. B. Garrod and P. G. Schyns (2012). “Perception-driven facial expression synthesis.” Computers & Graphics 36(3): 152-162.
Yuki, M., W. W. Maddux and T. Masuda (2007). “Are the windows to the soul the same in the East and West? Cultural differences in using the eyes and mouth as cues to recognize emotions in Japan and the United States.” Journal of Experimental Social Psychology 43(2): 303-311.
It seems to me that the problem has been that an arbitrary list was initially assigned by Darwin as an interm model, but it has been taken as a definitive model due to Darwin’s scientific status, but the emotion list was probably never intended as a definitive model.
In physics there are conservation of energy laws that keep intricate track of energy interactions as a dynamic function of opposites, positive and negative charges or color charge for example, for every action a reaction.
It seems to me emotion is very much a dynamic system, not a static system, emotions come in opposing pairs and also in more complex dynamic relationships like color charge. This suggests a simple list will not suffice, that like relativity it is the relationship between emotions that need be illistrated, emotions can be broken down still further, for example it is my contention that “Happy” or “sad” are component of emotion but they do not describe an emotional state.
The elements of the periodic table are like this, heavy or light, metallic or organic, simple or complex, high energy or low, these words do not describe “elements” but the elements of elements, the “trends” that relate the elements together into a practical system by which to understand how the elements relate to one another.
Originally the periodic table was also a partial list, modern chemistry began with the invention of the periodic table because only the table could reveal the dynamic trends (relationships) between the elements.
We need the same kind of dynamic table only for the emotional elements which I suspect are fewer, more like as if we considered elemental families one element. I have a purposed system of emotional dynamics that seems to tie in quite well to the evidence of how facial expression and music of voice communicate emotion based on clues gained through my array system musical instruments such as the patented array keyboard
https://www.google.com/patents/US6501011